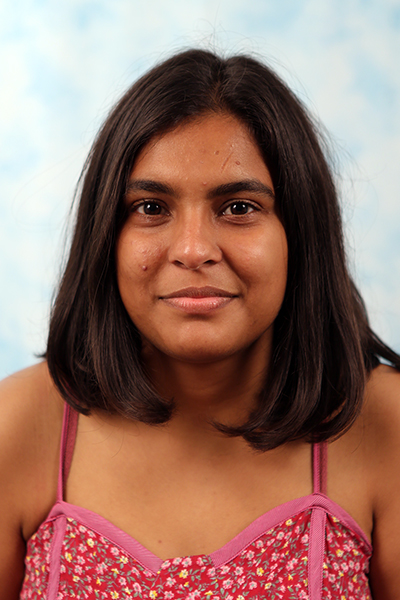
Carnegie Mellon University
12:00 pm to 1:00 pm
TBA
Zoom Link: https://cmu.zoom.us/j/96397153508
Title: A Comprehensive Study of Unsupervised Classification Techniques for Hyperspectral Datasets
Abstract:
Unsupervised learning and in this specific research, clustering regional composition in hyperspectral images, poses significant challenges in the fields of machine learning and remote sensing. Hyperspectral images capture the spectral information in many wavelengths, as opposed to typical images that only capture three: red, blue, and green. They are high-dimensional and have considerable noise, and class overlap which add to the difficulty in experimentation, analysis, and interpretation. This research conducts a comprehensive study of clustering techniques when applied to hyperspectral images. We focus on finding answers to some of the open questions present in the literature. We look at clustering techniques in terms of theoretical, algorithmic, and empirical differences. We evaluate the impact of changes in spectral and spatial resolution, and the number of classes present in the data. We also perform a hyperparameter analysis of dimensionality reduction techniques. We observe that spatial information along with spectral information is important for clustering. It is also imperative to note that no one algorithm is applicable to all datasets and this remains an open question too.
Committee:
David Wettergreen, chair
Ioannis Gkioulekas
Alberto Candela