
MSR Thesis Defense
December
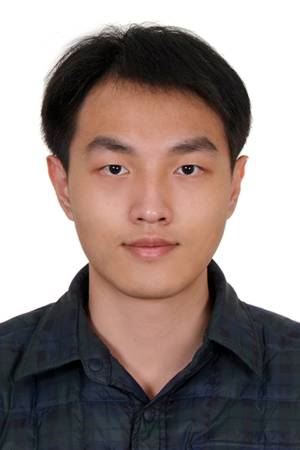
Abstract:
Deep generative models make visual content creation more accessible to novice users by automating the synthesis of diverse, realistic content based on a collected dataset. However, the current machine learning approaches miss several elements of the creative process — the ability to synthesize things that go far beyond the data distribution and everyday experience, and a user-friendly interface to create or edit a model easily.
To begin to address this issue, we enable a user to “warp” a given model by editing just a handful of original model outputs with desired geometric changes. Our method updates the model weights with a reconstruction loss on edited examples. Furthermore, to combat overfitting, we propose a latent space augmentation method based on style-mixing. Our method allows a user to create a model that synthesizes endless objects with defined geometric changes, enabling the creation of a new generative model without the burden of curating a large-scale dataset.
Next, we present a method for rewriting GANs with one or more sketches. We change the weights of an original GAN model according to user sketches. We encourage the model’s output to match the user sketches through a cross-domain adversarial loss, and we explore different regularization methods to preserve the original model’s diversity and image quality. Experiments have shown that our method can mold GANs to match shapes and poses specified by sketches while maintaining realism and diversity.
Committee:
Jun-Yan Zhu
Shubham Tulsiani
Yufei Ye