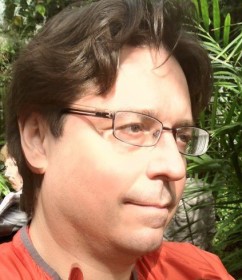
9:30 am to 12:00 am
Event Location: GHC 6501
Abstract: An iterative model-based method is proposed for improving linguistic structure, segmentation, and prosodic annotations that correspond to the delivery of each utterance as regularized across the data. For each iteration, the training utterances are resynthized according to the existing symbolic annotation. Values of various features and subgraph structures are “twiddled:” each is perturbed based on the features and constraints of the model. Twiddled utterances are evaluated using an objective function appropriate to the type of perturbation and compared with the unmodified, resynthesized utterance. The instance with least error is assigned as the current annotation, and the entire process is repeated. At each iteration, the model is re-estimated, and the distributions and annotations regularize across the corpus. As a result, the annotations have more accurate and effective distributions, which leads to improved control and expressiveness given the features of the model.
Committee:Alan W. Black, Chair
Jack Mostow
Alex Rudnicky
Julia Hirschberg, Columbia University