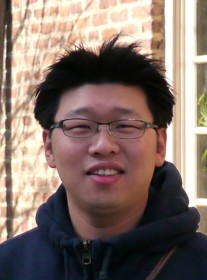
3:00 pm to 4:00 pm
Event Location: NSH 1507
Bio: Jonghyun Choi is a PhD candidate in the Computer Vision Lab at the University of Maryland, working with Prof. Larry Davis. He received the BS and MS degrees in electrical engineering and computer science from Seoul National University, Seoul Korea in 2003 and 2008 respectively. He has worked as an intern in various research labs including US Army Research Lab, Adobe Research, Disney Research Pittsburgh and Microsoft Research Redmond. His research interest includes visual category recognition using weakly labeled data, regularized classifier learning and improving the performance by external semantic information.
Abstract: Visual categories are defined by semantic concepts of objects, not by their appearance; for example, `tower’ or `beach’. Unlike specific object classes such as `Eiffel Tower’, the visual categories contain visually diverse sets of instances. Building a well-performing classifier for visual categories requires a high level of generalization. To address the challenge, we propose two different approaches to build the generalizable classifiers exploiting diversity and commonality of given labeled samples; one is to expand the training set by augmenting unlabeled samples from a pool of images or videos and the other is to divide the categories into subcategories and build an ensemble of classifiers that share category-wide commonality. We improve classification accuracy in a challenging ‘few-shot’ learning scenario.