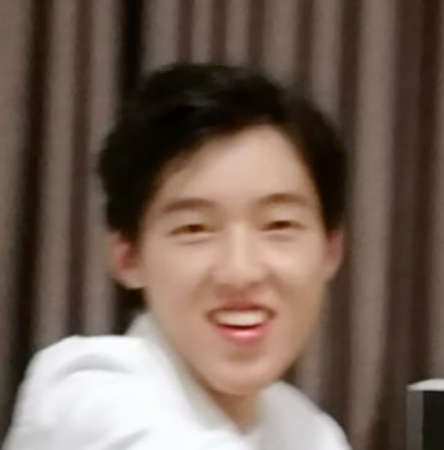
Abstract:
Deploying robots in open-ended unstructured environments such as homes has been a long-standing research problem. However, robots are often studied only in closed-off lab settings, and prior mobile manipulation work is restricted to pick-move-place, which is arguably just the tip of the iceberg in this area. In this thesis, we introduce the Open-World Mobile Manipulation System, a full-stack approach to tackle realistic articulated object operation, e.g. real-world doors, cabinets, drawers, and refrigerators in open-ended unstructured environments. The robot utilizes an adaptive learning framework to initially learn from a small set of data through behavior cloning, followed by learning from online practice on novel objects that fall outside the training distribution. We also develop a low-cost mobile manipulation hardware platform capable of safe and autonomous online adaptation in unstructured environments with a cost of around 25,000 USD. In our experiments, we utilize 20 articulate objects across 4 buildings in an university campus. With less than an hour of online learning for each object, the system is able to increase the success rate from 50% of BC pre-training to 95% using online adaptation.
Committee:
Professor Deepak Pathak (Chair)
Professor Guanya Shi
Kenneth Shaw