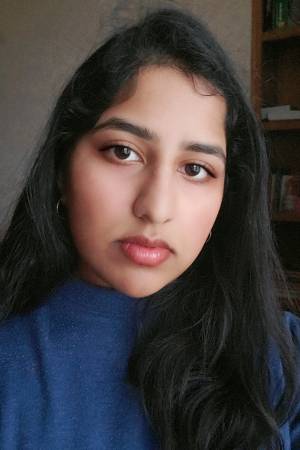
Abstract:
In the field of aviation, the Detect and Avoid (DAA) problem deals with incorporating collision avoidance capabilities into current autopilot navigation systems. In order to standardize DAA capabilities, ASTM has published performance requirements to define safe DAA operations of unmanned aircraft systems (UAS). However, the performance of DAA models are entirely dependent on the distribution that models are evaluated on and do not generalize well to other lighting and weather conditions not found in the training distribution. In this work, we analyze the current state of the art model for DAA and determine its sensitivity to changes in the operational data distribution. We also explore both test time data transformations and robust training techniques to improve the generalizability of the model. With strong assumptions, we show that these methods can improve model recall by up to 7x. However, with assumptions reflective of real world deployment scenarios, they provide marginal benefits. In the future, we will explore both out of distribution detection as well as generative methods for improving robustness.
Committee:
Sebastian Scherer, Chair
Lujo Bauer
Henny Admoni
Shivam Vats