
RI Seminar
February
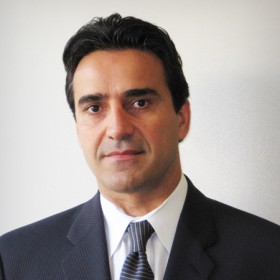
3:30 pm to 12:00 am
Event Location: NSH 1305
Bio: Dr. Azim Eskandarian is a Professor of Engineering and Applied Science at The George Washington University (GW). He has been the founding director of the Center for Intelligent Systems Research (CISR) since 1996 and the director of the “Transportation Safety and Security” program since 2002, which is one of GW’s competitively selected major Areas of Excellence. He was also the co-founder of the National Crash Analysis Center (NCAC) in 1992 and its director from 1998 to 2002. He has over 27 years of academic research and engineering experience in dynamics and control, intelligent systems, and applied mechanics, with applications in intelligent vehicles, vehicle dynamics and control, automotive and transportation safety, and robotics. Prior to joining GW in 1993, he was an assistant professor at Pennsylvania State University (1989-92) and earlier worked as an engineer and project manager in industry (1983-89) in R&D and design for mechanical/structural systems of military vehicles.
Dr. Eskandarian has been instrumental in the establishment of a new and unique graduate program of study in Transportation Safety and Intelligent Transportation Systems at GW. He has published over 132 refereed journal (54) and conference (78) papers and three edited volumes and one book. His research has been covered in several media. He is an associate editor of IEEE ITS Transactions and International J. of Automotive Technology, and a board member of IMechE J. of Multi-body Dynamics, International J. of Vehicle Autonomous Systems, and International J. of Vehicle Information and Communications Systems. He has been elected to the Board of Governors of the IEEE ITS Society and is similarly active in ASME Dynamic Systems and Control and SAE technical committees. He has served by invitation on several government (DOT), NSF, and NAS committees and panels. He received his B.S. (with honors), M.S., and D.Sc. degrees in Mechanical Engineering from GWU, Virginia Polytechnic Institute and State University, and GWU in 1982, 1983, and 1991, respectively.
Abstract: Collision avoidance and integrated (passive and active) safety systems are required to comprehensively mitigate the vehicle crash problem. This talk specifically addresses the problem of drowsy driving. In the United States over 1,000 fatalities (from FARS 2007 data), 47,000 injuries (from NASS/GES 2007 data), and 80,000 crashes are annually caused by drowsiness and fatigue, indicating a serious vehicle safety problem. It is also believed that statistical data underestimate the true magnitude of the problem due to the difficulty and uncertainty in determining crash causation after the fact from accident scenes and collected data. Most drowsiness related crashes are of high severity due to the major loss of driver control ability. Standard lane-departure warning is not a sufficient countermeasure for drowsiness or fatigue related crashes because of anomalies in steering behavior, unpredictable state of drivers, and severe loss of control in these crashes. Therefore, methods of detecting drowsiness before the drivers’ onset of sleep are of critical importance.
A new detection method (algorithm) is developed which focuses solely on the steering wheel data to detect drivers’ drowsiness or fatigue condition. Experimental data from track and driving simulator studies have shown strong correlation between the level of drowsiness and the resulting irregularity in driver steering. Unlike physiological or facial and eye monitoring methods, this approach is totally unobtrusive. Our algorithm uses an Empirical Mode Decomposition of the steering angle signal to extract specific drowsiness-affected features. These signal features represent the steering control degradation phases of drowsy drivers. A pattern recognition scheme is devised to classify the measured features into alert or drowsy state. The method is independent of road geometry and lane markings and accounts for driver variability. Driving simulator experiments have been conducted using commercially licensed (truck) drivers. The algorithm has shown promising results with over 80% accuracy in detecting drowsiness. It also achieves detection sufficiently early prior to a hazardous condition (i.e. crashes or lane departures). The method has been licensed and a US patent application is pending.