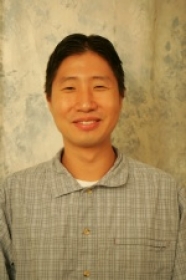
3:00 pm to 4:00 pm
Event Location: NSH 1109
Bio: Gunhee Kim is a postdoctoral researcher at Disney Research Pittsburgh. Prior to that, he received a Ph.D. degree at Computer Science Department of Carnegie Mellon University (CMU) in 2013, advised by Eric P. Xing. He earned a master’s degree under supervision of Martial Hebert in Robotics Institute, CMU in 2008. He also worked as a visiting student in Antonio Torralba’s group at CSAIL, MIT and Fei-Fei Li’s group at Stanford University. His principal research interest is solving computer vision and web mining problems that emerge from big visual data shared online, by developing scalable machine learning and optimization techniques.
Abstract: This is a joint work with Leonid Sigal and Eric P. Xing. In this paper, we address the problem of jointly summarizing large sets of Flickr images and YouTube videos. Starting from the intuition that the characteristics of the two media types are different yet complementary, we develop a fast and easily-parallelizable approach for creating not only high-quality video summaries but also novel structural summaries of online images as storyline graphs. The storyline graphs can illustrate various events or activities associated with the topic in a form of a branching network. The video summarization is achieved by diversity ranking on the similarity graphs between images and video frames. The reconstruction of storyline graphs is formulated as the inference of sparse time-varying directed graphs from a set of photo streams with assistance of videos. For evaluation, we collect the datasets of 20 outdoor activities, consisting of 2.7M Flickr images and 16K YouTube videos. Due to the large-scale nature of our problem, we evaluate our algorithm via crowdsourcing using Amazon Mechanical Turk. In our experiments, we demonstrate that the proposed joint summarization approach outperforms other baselines and our own methods using videos or images only.