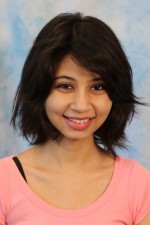
Abstract:
This thesis explores diverse decision-making strategies for autonomous agents by examining knowledge-dependent and data-dependent approaches in stationary and dynamic data environments. We address five core research problems across three thematic areas: knowledge-dependent, stationary data-dependent, and evolving data-dependent decision-making.
We first investigate knowledge-driven decision-making within robotic swarms, characterizing vulnerabilities in systems governed by consistent rule-following behaviors. Here, adversarial agents exploit predictable patterns in swarm actions, demonstrating the risks inherent in structured decision protocols.
The second theme shifts to stationary data, where we leverage expert demonstrations to inform decision-making. Two studies illustrate this: one develops socially intelligent robotic interactions through imitation learning, while the other unifies multiple methods of reward parameter estimation in expert policies under a common optimization framework. Together, these studies highlight how structured, stable examples can enhance model precision and generalization in social and reward-driven contexts.
The final focus is on continual learning to mitigate catastrophic forgetting in dynamic environments. We propose methods for incremental object detection, first by incorporating new object classes in images without destabilizing existing knowledge, and then extending this framework to videos in a domain-incremental setting. By leveraging temporal context, this approach aims to support models capable of adapting to varied, evolving environments, crucial for applications like autonomous driving and surveillance.
Thesis Committee Members:
Kris Kitani, Chair
Maxim Likhachev
Deva Ramanan
Hironobu Fujiyoshi, Chubu University