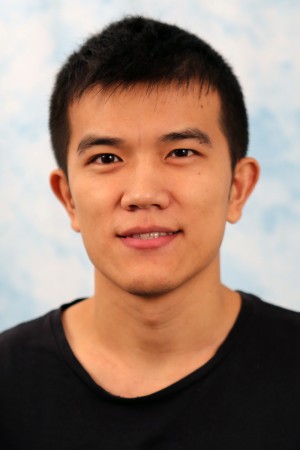
Abstract:
Scene reconstruction systems take in (3D) videos as input, and output 3D models with associated poses for inputs. With the demand of 3D content generation, the technique has been drastically evolving in recent years. For professionals equipped with depth sensors, efficient dense reconstruction systems have become available to efficiently recover scene geometry. For ordinary users with only monocular RGB images on affordable devices, real-life object reconstruction and novel view synthesis has become possible powered by neural rendering. However, large scale scene reconstruction from monocular videos is yet to be resolved.
In this thesis proposal, I will first revisit the state-of-the-art hierarchical large scale reconstruction frameworks for 3D videos. Specifically, I will discuss improvements I have accomplished in developing reliable and fast approaches for such systems: robust deep point cloud registration and efficient volumetric surface reconstruction. I will then propose to transfer the system to unposed monocular videos, enumerate challenges, and discuss potential solutions. The tentative contributions include: 1) a hybrid sparse scene representation for fragment surface reconstruction from RGB video segments; 2) robust fragment registration that enhances local surface consistency; and 3) hierarchical fragment fusion and pose refinement that ensures global consistency at a large scale. The proposed system will be mainly focusing on room-scale indoor scenes.
Thesis Committee Members:
Michael Kaess, Chair
Ji Zhang
Shubham Tulsiani
Vladlen Koltun, Apple