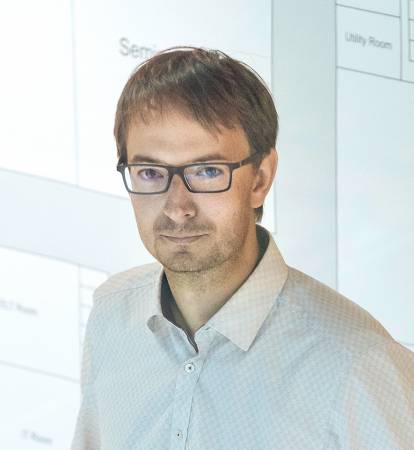
11:00 am to 12:00 pm
Virtual VASC Seminar:
https://cmu.zoom.us/j/96635002737?pwd=RkxGVlJaUTlhcDdGeVBPcnpTS015dz09
Abstract:
In this talk, I will show several recent results of my group on learning neural implicit 3D representations, departing from the traditional paradigm of representing 3D shapes explicitly using voxels, point clouds or meshes. Implicit representations have a small memory footprint and allow for modeling arbitrary 3D toplogies at (theoretically) arbitrary resolution in continuous function space. I will show the abilitiy and limitations of these approaches in the context of reconstructing 3D geometry (Occupancy Networks), texture (Texture Fields / Surface Light Fields) and motion (Occupancy Flow). I will further demonstrate Differentiable Volumetric Rendering (DVR) for learning implicit 3D models using only 2D supervision through implicit differentiation of the level set constraint. Finally, I will introduce GRAF, a generative 3D model for neural radiance fields which generates 3D consistent photo-realistic renderings from unstructured and unposed image collections of various objects.
BIO:
Andreas Geiger is professor at the University of Tübingen and group leader at the Max Planck Institute for Intelligent Systems. Prior to this, he was a visiting professor at ETH Zürich and a research scientist at MPI-IS. He studied at KIT, EPFL and MIT and received his PhD degree in 2013 from the KIT. His research interests are at the intersection of 3D reconstruction, motion estimation, scene understanding and sensory-motor control. He maintains the KITTI vision benchmark.
Speaker Homepage: www.cvlibs.net
Sponsored in part by: Facebook Reality Labs Pittsburgh