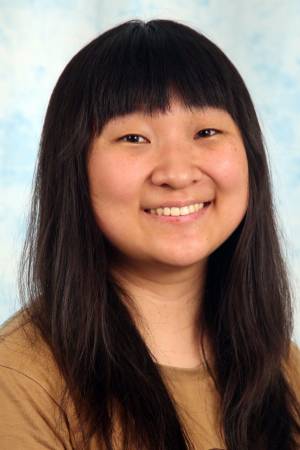
Learning Distributional Models for Relative Placement
Abstract: Relative placement tasks are an important category of tasks in which one object needs to be placed in a desired pose relative to another object. Previous work has shown success in learning relative placement tasks from just a small number of demonstrations, when using relational reasoning networks with geometric inductive biases. However, such methods fail to consider that demonstrations for the same task can be fundamentally multimodal, like a mug hanging on any of n racks. We propose a method that retains the provably translation-invariant and relational properties of prior work but incorporates additional properties that enable learning multimodal, distributional examples. We show that our method is able to learn precise relative placement tasks with a small number of multimodal demonstrations with no human annotations across a diverse set of objects within a category.
Committee:
David Held (Chair)
Oliver Kroemer
Shubham Tulsiani
Nikolaos Gkanatsios