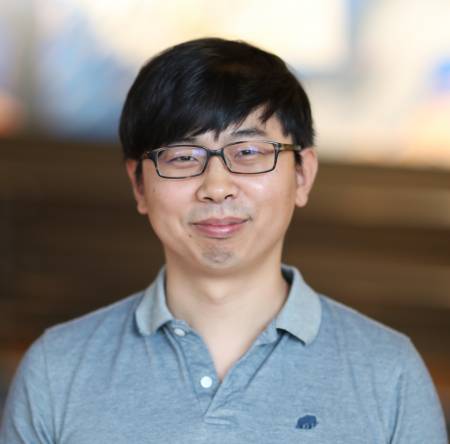
Abstract: Optical flow provides important information about the dynamic world and is of fundamental importance to many tasks. In this talk, I will present my work on different aspects of learning optical flow. I will start with the background and talk about PWC-Net, a compact and effective model built using classical principles for optical flow. Next, I will talk about AutoFlow, a simple and effective method to render training data for optical flow that optimizes the performance of a model on a target dataset. Finally, I will talk about learning articulated shape reconstruction from monocular videos using optical flow.
Bio: Deqing Sun is a staff research scientist and manager at Google working on computer vision and machine learning. He received a Ph.D. degree in Computer Science from Brown University. He served as an area chair for CVPR/ECCV/BMVC, and co-organized several workshops/tutorials at CVPR, ECCV, and SIGGRAPH. He is a recipient of the best paper honorable mention award at CVPR 2018, the first prize in the robust optical flow competition at CVPR 2018 and ECCV 2020, the PAMI Young Researcher award in 2020, and the Longuet-Higgins prize at CVPR 2020.
Homepage: https://deqings.github.io
Sponsored in part by: Facebook Reality Labs Pittsburgh