
PhD Thesis Proposal
December
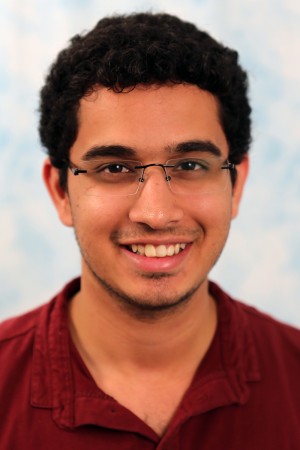
Abstract:
The growing need for fully autonomous aerial operations in shared spaces, necessitates the development of reliable agents capable of navigating safely and seamlessly alongside uncertain human agents. In response, we advocate endowing autonomous agents with the ability to predict human actions, comprehend and ground abstract rules in the action space, and embrace the uncertainty that is inherent in cooperative decision-making alongside humans.
We first start by developing data-driven trajectory prediction algorithms from historical data collected at local airports. Because vanilla imitation learning lacks the ability to explicitly distill underlying rules-of-the-road, we delve deeper into embedding rules as Signal Temporal Logic specifications into policy rollouts. We showcase that this augmentation enhances overall planning robustness. By amalgamating these techniques into a cooperative game framework, we present SoRTS, a novel social navigation algorithm. SoRTS leverages Monte Carlo Tree Search to facilitate long-term, socially conscious decision-making for collaborative agents. We perform user studies to showcase that SoRTS excels at navigating complex cooperative scenarios.
Towards the end, we will also discuss the limitations and propose future work on adaptive safety and fallback mechanisms to address unforeseen or unmodeled human behaviors. The thesis will also discuss various datasets and tools that can facilitate research endeavors within the realm of fully autonomous aviation.
Thesis Committee Members:
Sebastian Scherer, Chair
Jean Oh
Andrea Bajcsy
Hamsa Balakrishnan, Massachusetts Institute of Technology