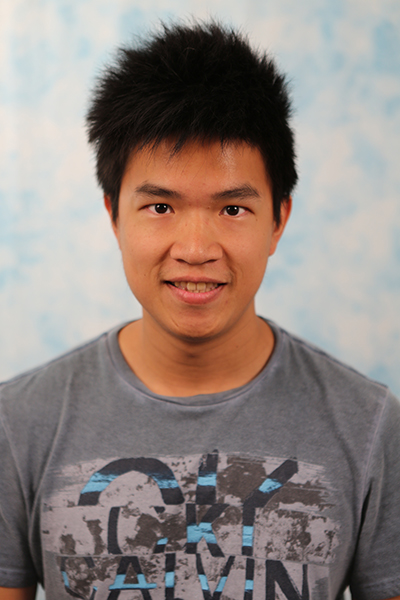
Carnegie Mellon University
2:00 pm to 3:00 pm
GHC 4405
Abstract:
Extracting geometric information from image data is a highly nonlinear problem that exhibits in a number of visual recognition tasks such as object localization, facial landmark tracking and human pose estimation. Successful alignment across image data often serves as a crucial component in making them possible. In this talk, I will present how one can achieve alignment for deep learning without the need of explicit geometric supervision. I will present three of my recent/previous works in this line: (1) resolving large spatial variations within image data for efficient visual learning, (2) correcting the geometry of images to create realistic composites, and (3) reconstructing 3D object meshes from RGB videos posed as a piecewise 2D image alignment problem, which can be achieved without any mask or depth information.
Research Qualifier Committee:
Simon Lucey, Advisor
Martial Hebert
Deva Ramanan
Chen Kong