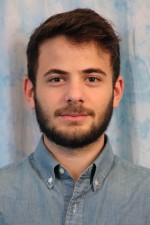
Carnegie Mellon University
11:30 am to 12:30 pm
NSH 3305
Abstract:
Reasoning about the future is fundamental to intelligence. In this work, I consider the problem of reasoning about the future actions of an intelligent agent. This poses two key questions. How can we build learning-based systems to forecast the behavior of observed agents (third-person, “allocentric forecasting”)? More challenging is the question: how should we build learning-based systems to forecast behavior of the systems themselves (first-person, or “egocentric forecasting”)? Throughout this work, I use demonstrations of agent behavior and often use rich visual data to drive learning.
Towards third-person, allocentric forecasting, I developed answers to excel in diverse, realistic, single-agent domains. These include sparse models to generalize from few demonstrations of human daily activity, adaptive models to continuously learn from demonstrations of human daily activity, and generative models learned from demonstrations of human driving behavior. Towards first-person, egocentric forecasting, i.e. forecasting a learning agent’s own behavior, I developed incentivized forecasting, which encourages an artificial agent to learn predictive representations in order to perform a task better.
While powerful and useful in these settings, our answers have only been tested in single agent domains. Yet, many realistic scenarios involve multiple agents undertaking complex behaviors: for instance, cars and people navigating and negotiating at intersections. Therefore, I propose to extend our generative framework to multiagent domains. In the allocentric setting, this involves generalizing representations and inputs to multiple agents. In the more difficult multiagent egocentric setting, our learning system should couple its forecasts of other agents with its own behavior. Another direction is to learn composable models, which would enable easier task transfer and greater reusability. Altogether, our answers will serve as a guiding extensible framework for further development of practical learning-based forecasting systems.
Thesis Committee Members:
Kris M. Kitani, Chair
Martial Hebert
Ruslan Salakhutdinov
Sergey Levine, University of California, Berkeley
Paul Vernaza, N.E.C. Labs America