
VASC Seminar
October
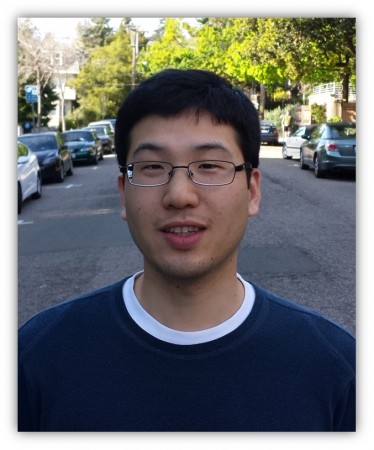
3:00 pm to 4:00 pm
GHC 6501
Abstract: Computer vision has made great strides for problems that can be learned with direct supervision, in which the goal can be precisely defined (e.g., drawing a box that tightly-fits an object). However, direct supervision is often not only costly, but also challenging to obtain when the goal is more ambiguous. In this talk, I will discuss our recent work on learning with indirect supervision. I will first present an approach that learns to focus on the relevant image regions given only indirect image-level supervision (e.g., an image tagged with “car”). This is enabled by a novel data augmentation technique that hides image patches randomly. I will then present an approach that learns to anonymize sensitive video regions while preserving activity signals in an adversarial framework. It accomplishes this by simultaneously optimizing for the indirectly-related task of misclassifying face identity and maximizing activity detection accuracy. We show that our anonymization method leads to superior performance compared to conventional hand-crafted anonymization methods including masking, blurring, and noise adding.
Bio: Yong Jae Lee is an Assistant Professor in the Department of Computer Science at the University of California, Davis. His research in computer vision and machine learning focuses on object recognition and video understanding. Before joining UC Davis in 2014, he spent one year as a post-doc at UC Berkeley and one year as a post-doc at Carnegie Mellon University, both under the supervision of Alyosha Efros. He received his PhD from the University of Texas at Austin in 2012 under the supervision of Kristen Grauman. He is a recipient of the Hellman Fellowship (2017), Army Research Office Young Investigator Award (2017), NSF CAREER Award (2018), and AWS ML Research Award (2018).
Homepage: http://web.cs.ucdavis.edu/~yjlee/