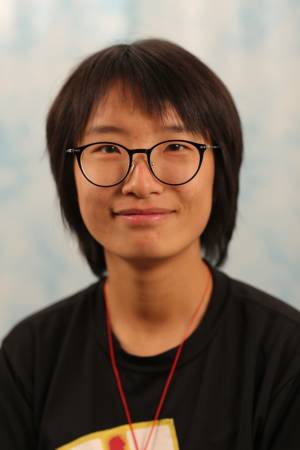
Abstract:
This thesis aims to develop a computer vision system that can understand everyday human interactions with rich spatial information. Such systems can benefit VR/AR to perceive the reality and modify its virtual twin, and robotics to learn manipulation by watching human. Previous methods have been limited to constrained lab environment or pre-selected objects with known 3D shape. This thesis explores learning general interaction priors from large-scale data that can generalize to novel everyday scenes for both perception and prediction.
The thesis is divided into two parts. The first part focuses on reconstructing interactions in 3D space for generic objects by leveraging hand-object interaction prior. The second part focuses on interaction prediction, including predicting spatial arrangements of human-object interactions and hallucinating possible interaction dynamics for scenes with multiple entities. The proposed work extends the pre-thesis works from single images to videos. We first present preliminary results of reconstructing interactions from everyday video clips. We propose a method to incorporate both multi-view signals and learning priors to understand every video clips that display heavy mutual occlusion and limited viewpoint variations. Then, we will discuss challenges and future works to scale interaction understanding from clips of a few seconds to videos of a few minutes.
Thesis Committee Members:
Shubham Tulsiani, Co-chair
Abhinav Gupta, Co-chair
Deva Ramanan
Angjoo Kanazawa, UC Berkeley
Andreas Geiger, University of Tübingen