
PhD Thesis Defense
August
20
Tue
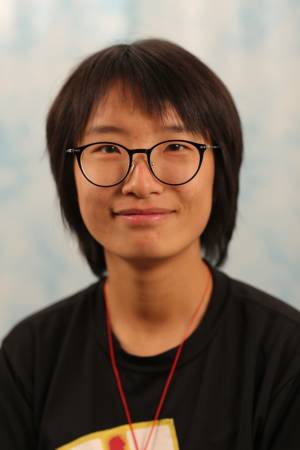
Learning to Perceive and Predict Everyday Interactions
Abstract:
This thesis aims to build computer systems to understand everyday hand-object interactions in the physical world – both perceiving ongoing interactions in 3D space and predicting possible interactions. This ability is crucial for applications such as virtual reality, robotic manipulations, and augmented reality. The problem is inherently ill-posed due to the challenges of one-to-many inference and the intricate physical interactions between hands and objects. To address these challenges, we explore a learning approach that mines priors from everyday data to enhance computer perception of interactions. Our goal is to develop methods for building 3D representations that respect the physical world’s inherent structure and can generalize to novel everyday scenes.
We first explore how to scale up 3D object priors for single-view object reconstruction in isolation, by introducing a learning technique for unsupervised, category-level 3D object reconstruction from unstructured image collections. Furthermore, we argue that interactions between hands and objects should not be marginalized as occlusion noise, but rather explicitly modeled to improve 3D reconstruction. To this end, we propose an approach to reconstruct hand-object interactions from a single image by leveraging hand pose information to better infer in-hand objects. Our research then extends the core idea to reconstruction from short video clips, where we combine multi-view cues with data-driven priors for accurate 3D inference. While perceiving ongoing interactions allows for predicting possible interactions, we also explore interaction synthesis – predicting spatial arrangements of human-object interactions. We propose a generative method that leverages a large-scale pre-trained model to achieve realistic, controllable, and generalizable predictions of novel everyday objects. Finally, this thesis presents a unified generative prior for hand-object interactions, allowing for both reconstruction and prediction tasks. We also make efforts to scale up the training data by aggregating multiple existing real-world interaction datasets. We demonstrate that the resulting joint prior can facilitate interaction reconstruction and prediction, outperforming current task-specific methods.
Thesis Committee Members:
Shubham Tulsiani, Co-Chair
Abhinav Gupta, Co-Chair
Deva Ramanan
Andreas Geiger, University of Tübingen
Angjoo Kanazawa, UC Berkeley