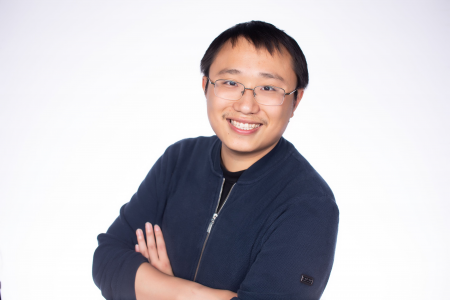
Abstract:
Video understanding has achieved tremendous success in computer vision tasks, such as action recognition, visual tracking, and visual representation learning. Recently, this success has gradually been converted into facilitating robots and embodied agents to interact with the environments. In this talk, I am going to introduce our recent efforts on extracting self-supervisory signals and 3D structure from videos and using this knowledge to help robots learn. I will first talk about our work on contrastive learning with videos, and how self-supervision in time can help Reinforcement Learning agents to continuously adapt to new environments (e.g., Sim2Real transfers). Going beyond RL, human videos also provide an efficient way for robots to learn by imitation. I will introduce our work on generalizing 3D hand-object pose and shape estimations, articulation modeling. By embedding these techniques, we propose a new Dexterous Manipulation from Videos (DexMV) benchmark on bridging 3D vision and imitation learning. The talk will not be recorded.
BIO:
Xiaolong Wang is an Assistant Professor of the ECE department at the University of California, San Diego. He is affiliated with the CSE department, Center for Visual Computing, Contextual Robotics Institute, and Artificial Intelligence Group. He received his Ph.D. in Robotics at Carnegie Mellon University. His postdoctoral training was at the University of California, Berkeley. His research focuses on the intersection between computer vision and robotics. He is particularly interested in learning visual representation from videos in a self-supervised manner and use this representation to guide robots to learn. Xiaolong is the Area Chair of CVPR, AAAI, ICCV. More details are available on his homepage: https://xiaolonw.github.io/
Homepage: https://xiaolonw.github.io/
Sponsored in part by: Facebook Reality Labs Pittsburgh