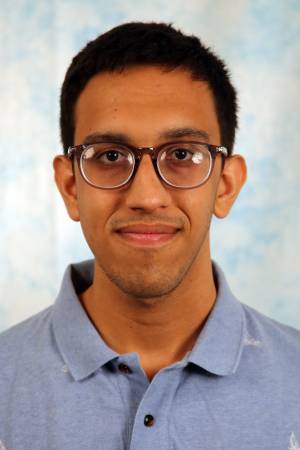
Abstract:
The inability to explore environments efficiently makes online RL sample-inefficient. Most existing works tackle this problem in a setting devoid of prior information. However, additional affordances may often be cheaply available at the time of training. These affordances include small quantities of demo data, simulators that can reset to arbitrary states and domain specific attributes. Such affordances can serve as valuable resources to guide exploration and help learn better policies faster.
We explore different ways to utilize such affordances to bootstrap online learning effectively. We first describe a method that re-parameterizes the action space through Bezier curves to induce an inductive bias towards natural vehicular trajectories, accelerating the learning of driving policies. Subsequently, we show that when provided with an arbitrarily resettable simulator and one demonstration trajectory, training with a suitable choice of an auxiliary start state distribution can significantly improve sample-efficiency.
Committee:Prof. Jeff Schneider (Advisor)
Prof. Katerina Fragkiadaki
Swaminathan Gurumurthy