
PhD Thesis Defense
February
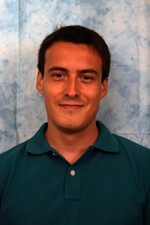
9:30 am to 10:30 am
GHC 6501
Tomas Simon
Carnegie Mellon University
Abstract
This thesis develops methods for social signal reconstruction—in particular, we measure human motion during social interactions. Compared to other work in this space, we aim to measure the entire body, from the overall body pose to subtle hand gestures and facial expressions. The key to achieving this without placing markers, instrumentation, or other restrictions on participants is the Panoptic Studio, a massively multi-view capture system which allows us to obtain 3D reconstructions of room-sized scenes.
To measure the position of joints and other landmarks on the human body, we combine the output of 2D keypoint detectors across multiple views and triangulate them in 3D. We develop a semi-supervised training procedure, multi-view bootstrapping, which uses 3D triangulation to generate training data for keypoint detectors. We use this technique to train fine-grained 2D keypoint detectors for landmarks on the hands and face, allowing us to measure these two important sources of social signals.
To model human motion data, we present the Kronecker Markov Random Field (KMRF) model for keypoint representations of the face and body. We show that most of the covariance in natural body motions corresponds to a specific set of spatiotemporal dependencies which result in a Kronecker or matrix normal distribution over spatiotemporal data, and we derive associated inference procedures that do not require training sequences. This statistical model can be used to infer complete sequences from partial observations and unifies linear shape and trajectory models of prior art into a probabilistic shape-trajectory distribution that has the individual models as its marginals.
Finally, we demonstrate full-body motion reconstructions by using the KMRF model to combine the various measurements obtained from the Panoptic Studio. We capture a dataset of groups of people engaged in social games and fit mesh models of the body, face, and hands—a representation that encodes many of the social signals that characterize an interaction and can be used for analysis, modeling, and animation.
Thesis Committee
- Yaser Sheikh, Co-chair
- Iain Matthews, Co-chair
- Fernando De la Torre
- Brooke Feeney
- David Fleet, University of Toronto