
RI Seminar
April
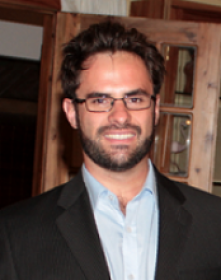
3:30 pm to 4:30 pm
Event Location: NSH 1305
Bio: Gabe Sibley is an Assistant Professor in Computer Science at the University of Colorado, Boulder. Before joining CU, Gabe was an Assistant Professor in Computer Science at George Washington University and Director of the Autonomous Robotics & Perception Lab. Previously, Gabe was a Junior Research Fellow at Somerville College, Oxford, and a post-doctoral research assistant in the Mobile Robotics Group of the Oxford University Engineering Department working with Professor Paul Newman. Gabe was a PhD student at the Robotic Embedded Systems Laboratory at the University of Southern California under the supervision of Professor Gaurav Sukhatme, and a Robotics Engineer in the Computer Vision Group at NASA-JPL under Dr. Larry Matthies. At NASA-JPL, Gabe worked on long-range data-fusion algorithms for planetary landing vehicles, unmanned sea vehicles and unmanned ground vehicles. Gabe is interested in robot perception and how it enables effective autonomous behavior. That is, the synthesis of perception, planning and control. He uses probabilistic perception algorithms and estimation theory that enable long-term autonomous operation of mobile robotic systems, particularly in unknown environments. He has experience with vision based, real-time localization and mapping systems, and is interested in fundamental understanding of sufficient statistics that can be used to represent the state of the world. His research uses real-time, embodied robot systems equipped with a variety of sensors — including lasers, cameras, inertial sensors, etc. — to advance and validate algorithms and knowledge representations that are useful for enabling long-term autonomous operation.
Abstract: This talk will cover recent advances in mobile perception, planning and control from the Autonomous Robotics and Perception Group (ARPG) at the University of Colorado, Boulder. We will discuss results ranging from scalable visual-inertial SLAM, dense 3D SLAM, semantic SLAM, unsupervised object discovery, photometric SLAM and how these can be tightly integrated using model predictive control for high-speed ground vehicles.