
PhD Thesis Proposal
May
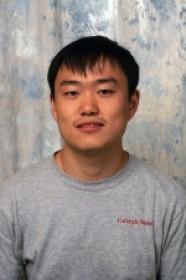
9:00 am to 12:00 am
Event Location: NSH 1305
Abstract: Non-rigid deformations of surfaces and volumes result in complex distortions in images. Modeling deformation can help better understand such real-world phenomena and extract useful information about the scenes. This is closely related to many core vision tasks, such as image alignment, matching, tracking, detection and recognition.
Modeling deformation remains challenging because deformation is nonlinear and the number of deformation parameters to be estimated is on the order of hundreds or even thousands. In this thesis, we propose both specific and generic deformation models for a variety of applications. Specific models use strong domain knowledge to facilitate deformation estimation; while general models are built on simple constraints and can be applied broadly. Two specific models are developed, one for rectification of document images to facilitate Optical Character Recognition (OCR), and the other for scene shimmering in turbulence medium useful to scene depth estimation.
For generic models, we have proposed Data-driven Descent (DDD) as a non-rigid image registration technique applied to water surface and cloth deformation, and a part-based Hierarchical Model applied to human pose estimation. The Data-driven Descent method is proven to obtain the globally optimal solution to the non-convex objective function used in deformation. Just like the Nyquist-Shannon theorem determines the number of samples needed to reconstruct the band-limited signal, Data-Driven Descent gives the required number of training samples for the globally optimal solution. In terms of performance, both Data-driven Descent and Hierarchical Model show strong empirical results that are better or on par with the state-of-art.
In proposed work, we will continue to analyze and extend the two generic models, Data-driven Descent and the Hierarchical model, to handle discontinuities in deformation such as occlusion and self-folding. We also seek a theoretical analysis of the hierarchical model and possible unification between the two generic models. In summary, this thesis aims to develop better deformation models and algorithms and provide novel datasets of deformation in various scenarios to spur new research.
Committee:Srinivasa G. Narasimhan, Chair
Martial Hebert
Fernando De la Torre
Kyros Kutulakos, University of Toronto
C. Lawrence Zitnick, Microsoft Research