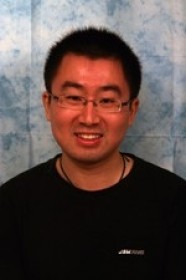
10:00 am to 12:00 am
Event Location: NSH 1305
Abstract: Brain-machine interfaces (BMIs) are a powerful class of assistive devices that may one day restore movement ability to paralyzed individuals. These devices act by creating a direct mapping between recorded neural activity and the movement of an external actuator, like a computer cursor or a robotic arm, bypassing defective neural transmission and muscle activation. Typical approaches to BMI algorithm design treat the system as if it were passive. That is, the relationship between neurons and the movement is constant and estimated from a single calibration session. However, brain-in-the-loop control systems are adaptive: parameters may change due to either recording instabilities or because of subject learning and neural plasticity. For my dissertation, I propose three separate, yet related approaches to better characterize and model brain-in-the-loop control systems.
In the first aim, we focus on combating recording instability. We build a model of parameters changes stemmed from recording noise and develop a dual estimation procedure to adaptively capture such changes. In the other two aims, we focus on subject learning in BMI control. In the second aim we try to study how we can provide assistance to hasten learning. We model the subject learning process and use it to derive enhanced training schedules to improve learning speed. In the third aim, we study what kind of BMI system should we provide the subject to control if we assume the subject can learn whatever system we give it. We model the BMI system design from the control theory perspective and develop a optimal plant design method to obtain the provably-optimal poster learning BMI system.
Committee:Steve M. Chase, Chair
Robert E. Kass
J. Andrew Bagnell
Patrick J. Loughlin, University of Pittsburgh