
This event has passed.
MSR Thesis Defense
July
20
Thu
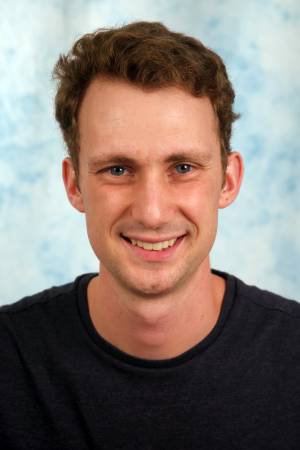
MSR Thesis Talk: David Russell
Title: Using Drones and Remote Sensing to Understand Forests with Limited Labeled Data
Abstract:
Abstract:
Drones and remote sensing can provide observations of forests at scale, but this raw data needs to be interpreted to further scientific understanding and inform effective management decisions. This thesis studies two problems under the realistic constraint of limited domain-specific
training data: tree detection for understanding carbon sequestration and vegetation mapping for forest fire mitigation. For tree detection, we process the drone data using structure from motion and align it to remote sensing imagery. Then, we compare different strategies using a deep learning detector on drone and remote sensing imagery with limited training data. For vegetation mapping, we localize fuel that causes forest fires by coupling image-based semantic segmentation trained on very few examples and LiDAR-based geometric reasoning. Finally, we introduce RAPTORS, a novel algorithm that plans where to collect sparse drone observations based on existing remote sensing data. We show that training a remote sensing-based vegetation classification model on observations from RAPTORS is more accurate than training on observations from a coverage-based approach, especially for rare classes. Overall, these experiments show how using machine learning, data harmonization across scales, and intelligent sampling can advance automated forest understanding with limited training data.
training data: tree detection for understanding carbon sequestration and vegetation mapping for forest fire mitigation. For tree detection, we process the drone data using structure from motion and align it to remote sensing imagery. Then, we compare different strategies using a deep learning detector on drone and remote sensing imagery with limited training data. For vegetation mapping, we localize fuel that causes forest fires by coupling image-based semantic segmentation trained on very few examples and LiDAR-based geometric reasoning. Finally, we introduce RAPTORS, a novel algorithm that plans where to collect sparse drone observations based on existing remote sensing data. We show that training a remote sensing-based vegetation classification model on observations from RAPTORS is more accurate than training on observations from a coverage-based approach, especially for rare classes. Overall, these experiments show how using machine learning, data harmonization across scales, and intelligent sampling can advance automated forest understanding with limited training data.
Committee:
Prof. David Wettergreen (advisor)
Prof. George Kantor
Prof. Marija Popović (University of Bonn)
Kshitij Goel
Kshitij Goel
Zoom Link: https://cmu.zoom.us/j/
Meeting ID: 939 5008 9058
Passcode: 770254