
MSR Thesis Defense
July
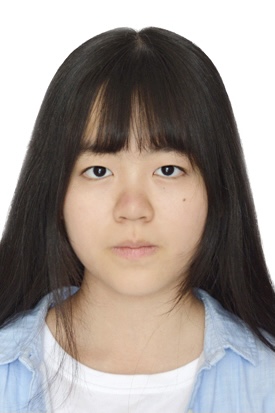
Title: On Generalization and Benchmarking on Physical Robots
Abstract:
Robotics research has seen significant advancements; however, the field remains predominantly demo-driven, making direct comparisons between methods difficult without replicating them on individual setups. While many simulation benchmarks exist, they usually feature contrived datasets and do not accurately reflect real-world performance. In my thesis, we address these challenges by proposing a more realistic data assumption for physical robots. We evaluate the generalization capabilities of offline reinforcement learning and imitation learning methods within this framework. We also introduce an initiative towards establishing a real-robot benchmark. Initial results from the benchmark and the launch of a NeurIPS competition highlight the feasibility of such systems.
Committee:
Prof. Abhinav Gupta (advisor)
Prof. David Held
Sudeep Dasari
ZOOM Link: https://cmu.zoom.us/j/