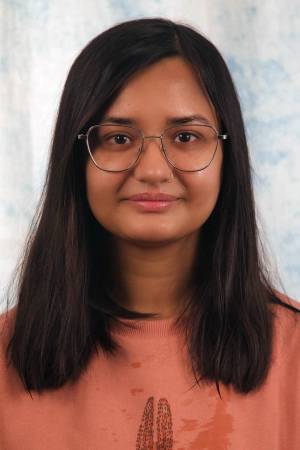
First, given the uncurated nature of long, untrimmed, continuous videos, learning effective representations require focusing on moments in time when interactions take place. Second, visual representations of daily activities should be sensitive to changes in the state of the object and the environment. However, current successful multi-modal learning frameworks encourage representations that are invariant to time and object states. We propose a self-supervised algorithm to learn representations from egocentric video data using multiple modalities of video and audio. To address the above challenges, we leverage audio signals to identify moments of likely interactions which are conducive to better learning. Motivated by the observation of a sharp audio signal associated with an interaction, we propose a novel self-supervised objective that learns from audible state changes caused by interactions. We validate these contributions extensively on two large-scale egocentric datasets, EPIC-Kitchens-100 and Ego4D, and show improvements on several downstream tasks, including action recognition, long-term action anticipation, object state change classification, and point-of-no-return temporal localization.
Prof. Abhinav Gupta (advisor)
Prof. David Held
Prof. Shubham Tulsiani
Yufei Ye