
MSR Speaking Qualifier
August
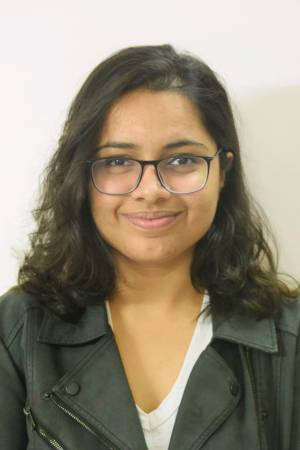
Title: Detecting Invisible People
Abstract:
Monocular object detection and tracking have improved drastically in recent years, but rely on a key assumption: that objects are visible to the camera. Many offline tracking approaches reason about occluded objects post-hoc, by linking together tracklets after the object re-appears, making use of reidentification (ReID). However, online tracking in embodied robotic agents (such as a self-driving vehicle) fundamentally requires object permanence, which is the ability to reason about occluded objects before they re-appear. In this work, we re-purpose tracking benchmarks and propose new metrics for the task of detecting invisible objects, focusing on the illustrative case of people. We demonstrate that current detection and tracking systems perform dramatically worse on this task. We introduce two key innovations to recover much of this performance drop. We treat occluded object detection in temporal sequences as a short-term forecasting challenge, bringing to bear tools from dynamic sequence prediction. Second, we build dynamic models that explicitly reason in 3D from monocular videos without calibration, using observations produced by monocular depth estimators. To our knowledge, ours is the first work to demonstrate the effectiveness of monocular depth estimation for the task of tracking and detecting occluded objects. Our approach strongly improves by 11.4% over the baseline in ablations and by 5.0% over the state-of-the-art in F1 score.
Committee:
Deva Ramanan (advisor)
Simon Lucey
Katerina Fragkiadaki
Achal Dave
Zoom Link: https://cmu.zoom.us/j/99155936991?pwd=OERQWThxUzJ6N0V4Ny9JcDVGSGVRUT09
Meeting ID: 991 5593 6991
Passcode: 720498