
MSR Speaking Qualifier
August
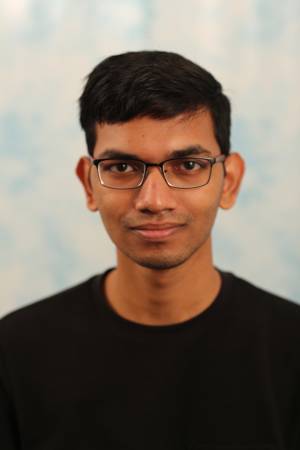
ZOOM Link: https://cmu.zoom.us/j/2388465851
Meeting ID: 238 846 5851
Title: Adaptive and Efficient Models for Intent Recognition
Abstract:
Assistive robots should have the ability to understand the intent of humans, predict their behavior, and plan to provide anticipatory assistance in complex real-life environments. In this thesis, we present adaptive and efficient algorithms for recognizing human intent.
We develop adaptive models for human intent recognition in a simulated search and rescue scenario. Humans vary widely in their behavior style due to different preferences, initial beliefs, internal world models, and planning mechanisms. A generic (non-adaptive) prediction model, therefore, has limited utility in this setting. Our adaptive model can recognize a rescuer’s behavior patterns online and make better predictions. We show that adaptive models trained on a wide variety of simulated planning-based agents can transfer to humans and outperform generic models trained on limited human data.
We also present an efficient inverse reinforcement learning algorithm, called f-IRL, which directly optimizes a parameterized reward function to match the demonstrator’s state distribution. We show that f-IRL can efficiently learn the demonstrator’s intent – it can learn to imitate control policies from just a single demonstration. In addition, we show that the learned reward can be used to transfer policies to different dynamics.
Committee:
Katia Sycara (advisor)
Changliu Liu
Wenhao Luo