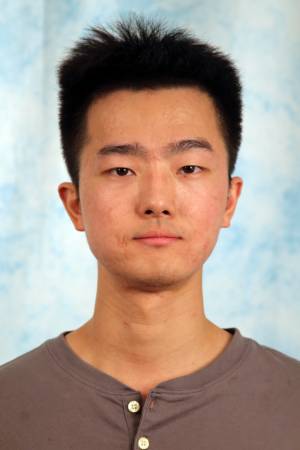
Abstract: This thesis introduces a novel transfer learning framework for deep reinforcement learning. The approach automatically combines goal-conditioned policies with temporal contrastive learning to discover meaningful sub-goals. The approach involves pre-training a goal-conditioned agent, finetuning it on the target domain, and using contrastive learning to construct a planning graph that guides the agent via sub-goals. Experiments on PointMaze and multi-agent coordination Overcooked tasks demonstrate improved sample efficiency, the ability to solve sparse-reward and long-horizon problems, and enhanced interpretability compared to baselines. The results highlight the effectiveness of integrating goal-conditioned policies with unsupervised temporal abstraction learning for complex multi-agent transfer learning.
Committee:
Katia Sycara (advisor)
Andrea Bajcsy
Sha Yi