
MSR Speaking Qualifier
July
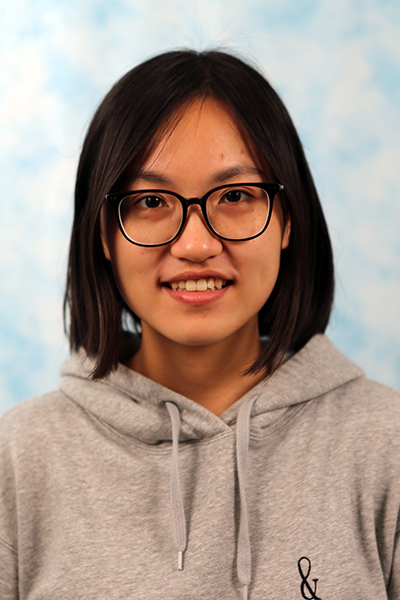
3:00 pm to 4:30 pm
NSH 4305
Title: Data-Efficient Stage Classification and Failure Detection for Robotic Screwdriving
Abstract:
Screwdriving is one of the most common assembly methods, yet its full automation is still challenging, especially for small screws. A critical reason is that existing techniques perform poorly in process monitoring and failure prediction. In addition, most solutions are essentially data-driven, thereby requiring lots of training data and laborious labeling. Moreover, they are not robust against varying environment conditions and suffer from generalization issues. To this end, we propose a stage and result prediction framework that combines knowledge-based process models with a hidden Markov model. The novelty of this work is the incorporation of operation-invariant characteristics such as screwdriving mechanics and stage transition graph, enabling our system to generalize across different experimental settings and largely reduce the required data and labeling. In our experiments, a system trained on M1.4×4 screws adapted with very little non-labeled data to three other screws (M1.2×3, M2.5×5, and M1.4×4) with widely varying tightening current, motor velocity, insertion force, and tightening force.
Committee:
Matthew T. Mason
Ralph Hollis
Ankit Bhatia