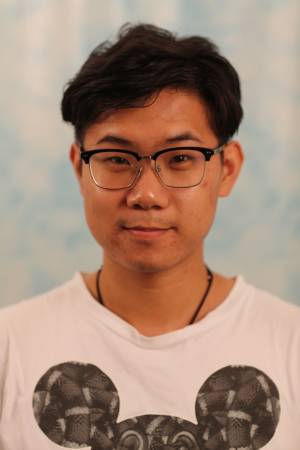
Title: Introducing Generative Models to Facilitate Multi-Task Visual Learning
Abstract:
Motivated by multi-task learning of shared feature representations, this talk considers a novel problem of learning a shared generative model that can facilitate multi-task learning. We present two systems to utilize generative modeling for other visual tasks. The first system focuses on learning a generative model for the joint task of few-shot recognition and novel-view synthesis with a feedback-based architecture. The other system contains a general multi-task oriented generative modeling (MGM) framework that leverages a generative network to facilitate multi-task visual learning. The motivations, architecture details, the experimental verifications, and the broader impact of the two systems will be demonstrated in detail in the talk.
Committee:
Martial Hebert (Advisor)
Yu-Xiong Wang (Advisor)
Jun-Yan Zhu
Nadine Chang
https://cmu.zoom.us/j/95995474117?pwd=eTVmcUtSbzkvWFp1VVB3Z0pQdlZIQT09p