
PhD Thesis Proposal
November
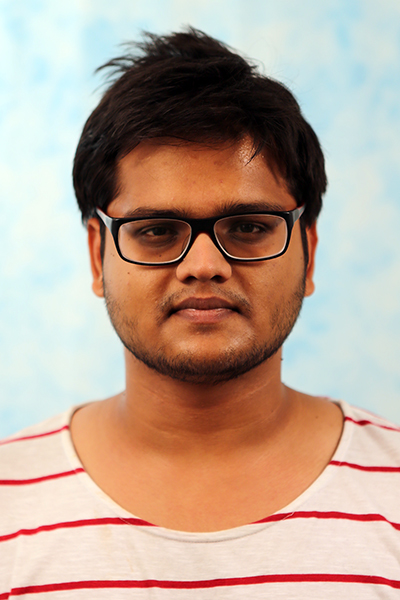
Abstract:
We study the problem of multi-human 3D reconstruction from RGB videos captured in the wild. Humans have dynamic motion, and reconstructing them in arbitrary settings is key to building immersive social telepresence, assistive humanoid robots, and augmented reality systems. However, creating such a system requires addressing fundamental issues with previous works regarding the data and model architectures. In this thesis, we develop a large-scale 3D benchmark that evaluates multi-human reconstruction under challenging settings and top-down algorithms robust to occlusion and crowding.
Data – Obtaining 3D supervision at scale for our deep learning models is essential to generalize to the real world. However, unlike the large-scale 2D datasets, the diversity of the 3D datasets is significantly limited – primarily because manually annotating in the 3D space is impractical. As a result, popular 3D benchmarks are constrained to indoor environments or, at most, two human subjects if outdoors, stationary/slow camera motion, with limited occlusion. Therefore, we investigate using 3D synthetic data and construct a real multi-human 3D dataset that includes dynamic human activities and rapid camera motion neglected by earlier benchmarks to highlight the critical limitations of the existing methods.
Algorithm – A general multi-human 3D reconstruction method should be robust to scale variations and occlusions and incorporate absolute depth understanding. We introduce algorithms with these traits in 2D and 3D settings, which reason about multiple humans in dynamic environments and crowding. Our top-down approach exploits spatial-contextual information to reason about severely occluded humans in the 3D scene.
We plan to combine the two paradigms for the proposed work by leveraging our large-scale video data to build a global occlusion-aware 3D model robust to rapid-camera motion. One challenge is that dynamic cameras make it difficult to estimate human motions in consistent global coordinates. Another challenge comes from severe and long-term occlusions of humans, which can be caused by missed detection or complete obstruction by
objects and other people. Lastly, we explore feed-forward inference for high-resolution human digitization from videos.
Thesis Committee Members:
Kris Kitani, Chair
Deva Ramanan
Shubham Tulsiani
Angjoo Kanazawa, UC Berkeley