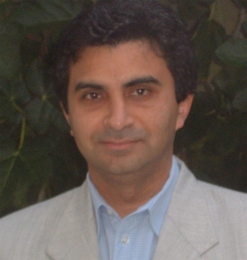
3:30 pm to 12:00 am
Event Location: NSH 3305
Bio: Milind Tambe is a Professor of Computer Science at University of Southern California(USC). He received his Ph.D. from the School of Computer Science at Carnegie Mellon University. He leads the TEAMCORE Research Group at USC, with research interests in multi-agent systems. He is a fellow of AAAI and recipient of the ACM/SIGART Agents Research award. He is also recipient of a special commendation from the city of Los Angeles given by the Los Angeles World Airport police, USC Viterbi School of Engineering Use-inspired research award, Okawa foundation faculty research award, special certificate of recognition from DHS University programs, and the ACM recognition of service award; and his papers have been selected as best papers or finalists for best papers at a dozen premier agents conferences and workshops including AAMAS and RoboCup.
Abstract: How do we build multiagent systems? Today, within the agents and multiagent systems community, we see four main approaches: logic-based belief-desire-intention (BDI), decision-theory and its incarnation in distributed markov decision problems (distributed MDPs or POMDPs), distributed constraint optimization (DCOPs) and finally, mechanism design or game-theoretic approaches. While there is exciting progress, we still lack sufficient testing of our theories in complex multiagent domains, to evaluate their promised strengths and uncover unanticipated limitations.
In this context, I will outline lessons learned in the Teamcore group’s recent efforts to transition theory into practice. I will first focus on research for randomizing plans for security applications, to avoid predictability that may be exploited by an opponent. This research has led to novel efficient algorithms for obtaining optimal mixed strategies in Bayesian Stackelberg games. Our optimal Stackelberg solvers are at the heart of ARMOR, a software scheduler that randomizes police checkpoints and canine patrols, actually deployed at the Los Angeles International Airport since August 2007. I will outline lessons learned from this deployment as well as in applying our Stackelberg solvers for randomizing placement of Federal Air Marshals on flights, and also from playing thousands of games against USC students. Next I will provide an overview of DCOP algorithms, and in particular the locally optimal “k-optimal” algorithms, that provide quality guarantees. An important missing step for DCOPs is transitioning to a complex multiagent domain; our application of DCOPs on a mobile sensor net calls into question key assumptions in DCOPs including their performance evaluation metrics. I will end the presentation with an overview of some key areas of current research, in particular providing an overview of recent research in distributed POMDPs, and efforts to transition these to complex real-world domains.