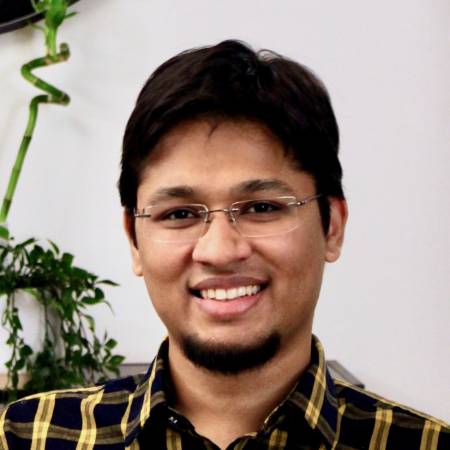
Monday, May 15
3:30 pm to 4:30 pm
3305 Newell-Simon Hall
3:30 pm to 4:30 pm
3305 Newell-Simon Hall
Navigating to Objects in the Real World
Abstract: Semantic navigation is necessary to deploy mobile robots in uncontrolled environments like our homes, schools, and hospitals. Many learning-based approaches have been proposed in response to the lack of semantic understanding of the classical pipeline for spatial navigation, which builds a geometric map using depth sensors and plans to reach point goals. Broadly, end-to-end learning approaches reactively map sensor inputs to actions with deep neural networks, while modular learning approaches enrich the classical pipeline with learning-based semantic sensing and exploration. But learned visual navigation policies have predominantly been evaluated in simulation. How well do different classes of methods work on a robot? We present a large-scale empirical study of semantic visual navigation methods comparing representative methods from classical, modular, and end-to-end learning approaches across six homes with no prior experience, maps, or instrumentation. We find that modular learning works well in the real world, attaining a 90% success rate. In contrast, end-to-end learning does not, dropping from 77% simulation to 23% real-world success rate due to a large image domain gap between simulation and reality. For practitioners, we show that modular learning is a reliable approach to navigate to objects: modularity and abstraction in policy design enable Sim-to-Real transfer. For researchers, we identify two key issues that prevent today’s simulators from being reliable evaluation benchmarks – (A) a large Sim-to-Real gap in images and (B) a disconnect between simulation and real-world error modes – and propose concrete steps forward.
Bio: Devendra Singh Chaplot is a Research Scientist at FAIR, Meta AI working at the intersection of Machine Learning, Computer Vision and Robotics. He has led the design of several AI systems which won the CVPR-2019 PointNav and CVPR-2020 ObjectNav, NeurIPS-2022 Rearrangement Habitat Challenges and the Visual-Doom AI Competition 2017. Chaplot is a recipient of the Facebook Fellowship Award and his research has received Best Paper and Best Demo awards at leading AI conferences. His research has also been featured in several popular media outlets such as MIT Technology Review, TechCrunch, Engadget, Popular Science, Kotaku, and Daily Mail. Chaplot received his Ph.D. in Machine Learning from Carnegie Mellon University and Bachelor’s degree in Computer Science from IIT Bombay.
Homepage: https://devendrachaplot.github.io/
Sponsored in part by: Meta Reality Labs Pittsburgh