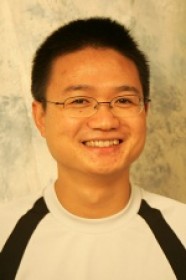
1:00 pm to 12:00 am
Event Location: NSH 1109
Abstract: This thesis tackles the problem of automatically discovering and modeling objects from a collection of images from the Activities of Daily Living (ADL) environment. I propose an approach that can discover object instances under severe clutter, occlusion, changes of view point, heterogeneity of object appearance and imperfect segmentation. The proposed approach exploits the regularity among instances of the same object seen in a pool of images and discover plausible object segments that are consistent as a group. Once such candidates of object instances are discovered, we use relational information between image segments to create hierarchical representations of object parts and their relationship. For each discovered object instance, we generate a 3D model using the collection of image segments that are associated with the object. Because the objects encountered in daily life are usually textureless and viewpoints are sparse, conventional 3D reconstruction techniques are not applicable. Instead, I propose an approach to generate 3D models of objects based on a sketch-based method. I demonstrate applications of the discovered object instances in categorizing objects that have similar use based on co-occurring and contextual information.
Committee:Takeo Kanade, Co-chair
Martial Hebert, Co-chair
Alexei A. Efros
Pietro Perona, California Institute of Technology
Jay Yagnik, Google Research