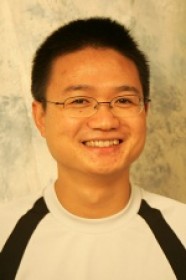
3:30 pm to 12:00 am
Event Location: NSH 1507
Abstract: This thesis tackles the problem of automatically discovering objects from a collection of images from the Activities of Daily Living (ADL) environment. We contribute, 1) a framework for discovering object instances under severe clutter, occlusion, changes of view point, heterogeneity of object appearance and imperfect segmentation; 2) a data-driven approach for discovering objects from sparse observations; 3) a data-driven approach to estimate the objectness of an image segment and to filter out segments that are less object-like, which improves the object discovery efficiency and quality.
The proposed object discovery framework exploits the regularity among instances of the same object seen in a pool of images and discover plausible object segments that are consistent as a group. Once such candidates of object instances are discovered, we use the group information to improve the initial imperfect segmentation.
Under this framework, finding the matches between different views is crucial. When each object is observed seamlessly from all of its possible viewpoints, such matches can be recovered by existing techniques. Yet the quality usually deteriorates when the observation is sparse. We propose a data-driven approach that transfers the metadata information among multi-views of product items to recover the links between different views of an object. We propose a way to build such database of product items using existing online services, such as Craigslist and Amazon. We develop “data-driven similarity” based on this object database. We show that this data-driven similarity is effective in finding matches between views of similar objects.
The unsupervised nature of the proposed framework means that one does not pose any prior on the input image segments, which are usually very “noisy”. Therefore, to increase the recall, a large number of initial image segments must be evaluated. This is very computationally expensive since the discovery program involves pairwise comparison between image segments. We introduce a data-driven approach that estimates the objectness of each input image segment and filter out the ones that are less object-like. Our approach simulates the decision making process of human cognition system and making use of 5 million images of products that a person might likely encounter in her daily life. We show that this data-driven approach significantly outperforms existing model-based approaches and improves object discovery performance.
Committee:Takeo Kanade, Co-chair
Martial Hebert, Co-chair
Alexei Alyosha Efros
Pietro Perona, California Institute of Technology
Jay Yagnik, Google