
PhD Speaking Qualifier
October
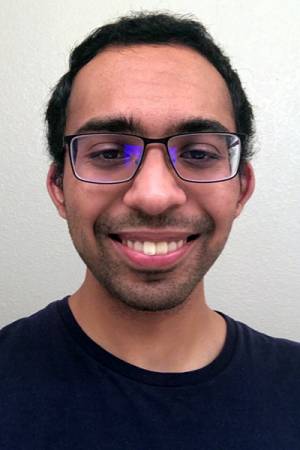
Abstract:
A standard critique of machine learning models (especially neural networks) is that they pick up on spurious correlations rather than causal relationships and are therefore brittle in the face of distribution shift. Solving this problem in full generality is impossible (i.e. there might be no good way to distinguish between the two). However, if we allow the learner to collect data via interaction with the surrounding environment, they can actually intervene on the system of interest through their actions and discover generalizable predictors. My research focuses on interactive learning of policies and models that do not suffer from spurious correlations and are therefore robust against induced distribution shifts. Using imitation learning as an example, I’ll be discussing how interaction with the environment can help us resolve issues of causation that result from 1) covariate shift, 2) temporally correlated noise, and 3) unobserved confounders.
Committee:
Drew Bagnell
Steven Wu
Zachary Lipton
Sudeep Dasari