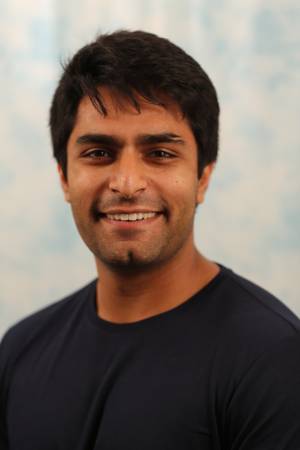
Abstract:
In many practical applications of reinforcement learning (RL), it is expensive to observe state transitions from the environment. For example, in the problem of plasma control for nuclear fusion, determining the next state for a given state-action pair requires querying an expensive transition function which can lead to many hours of computer simulation or dollars of scientific research. Such expensive data collection prohibits application of standard RL algorithms which usually require a large number of observations to learn. In this proposal, I address the problem of efficiently learning a policy from a relatively modest number of observations. The first section leverages ideas from Bayesian optimal experimental design to guide the selection of state-action queries for efficient learning. The second presents work which uses physical prior knowledge about the dynamics to more quickly learn an accurate model. Then, I give a brief overview of plasma control for nuclear fusion and comment on where I see opportunities for machine learning to improve the state of the art for physicists and engineers. I present initial work in this direction and give a plan for further experiments controlling the rampdown and flattop phases of a plasma shot both in simulation and on the DIII-D tokamak. Finally, I discuss my timeline for a thesis defense and the projects I would like to accomplish along the way.
Thesis Committee Members:
Jeff Schneider, Chair
Deepak Pathak
David Held
Stefano Ermon, Stanford University
Mark D. Boyer, Princeton Plasma Physics Laboratory