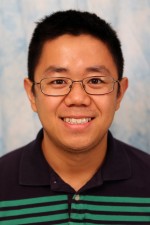
Carnegie Mellon University
2:00 pm to 3:00 pm
NSH 4305
Abstract:
Perception for autonomous robots presents a set of unique challenges: finding the right representation for 3D signals, adapting to an open-world setting, and exploiting geometric priors. Successfully detecting objects regardless of their labels lays a solid foundation for safe navigation. I will present two of my recent works in this line. First, I will talk about a new method for class-agnostic instance segmentation of LiDAR point clouds. By carefully mixing graph-theoretic algorithms with data-driven learning, this approach takes advantage of learning a 3D representation and ensures the output segmentation is optimal to a specific definition. I will also describe a simple approach to augment state-of-the-art voxel-based 3D object detectors with visibility recovered from raycasting.
Committee:
Deva Ramanan (Advisor)
David Held
Christopher Atkeson
Achal Dave