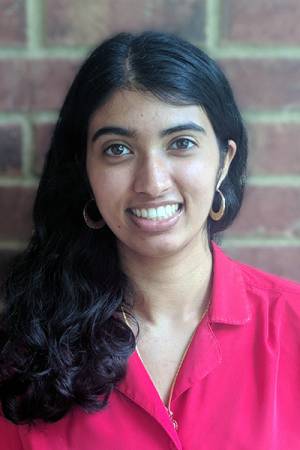
Personalized Context-aware Affective Nonverbal Robot Feedback
Abstract:
We first consider the problem of estimating context, specifically key features of the human state. We predict engagement-related events in an educational activity before the end of that activity, which could allow the robot to provide feedback early enough to improve the human’s experience. We then explore generating nonverbal affective robot behavior by correlating a simulated robot’s movements with displayed emotion. We develop a user study to show that matching the robot’s conveyed emotion with a matching affective movement has a positive impact on the human’s performance in a sorting game. Next we design a physical robot exercise coach as a platform where we can estimate context (exercise performance, fatigue level, etc.). With a user study, we examine the changes in perceptions and performance between different feedback styles for the robot. This provides us a basis on which to begin tailoring feedback styles to the individual. Finally, we propose new contributions to develop a personalized context-aware robot using a contextual bandit approach to dynamically adapt the robot’s feedback style to optimize both the human’s performance and experience. This brings together all the work presented in this thesis and aims to create a holistic framework for generating personalized context-aware nonverbal feedback that positively impacts the interaction with the human.
Thesis Committee Members:
Reid Simmons, Chair
Henny Admoni
Jean Oh
Sonia Chernova, Georgia Tech