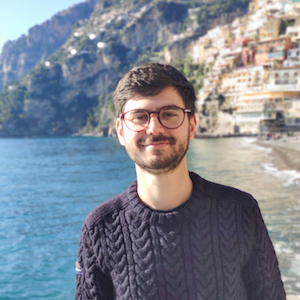
Fabio Pizzati
PhD student
Inria
Physics-informed image translation
- Abstract: Generative Adversarial Networks (GANs) have shown remarkable performances in image translation, being able to map source input images to target domains (e.g. from male to female, day to night, etc.). However, their performances may be limited by insufficient supervision, which may be challenging to obtain. In this talk, I will present our recent works on physics-informed image translation, showing that physical priors can be effectively leveraged to increase performances. I will first focus on CoMoGAN [1] where weak physical guidance with naive models is an effective signal for discovering continuous transformations on datasets without annotations. Then, I will introduce how realistic physical models can be exploited for disentangled image generation [2][3].
[1] F. Pizzati et al., CoMoGAN: continuous model-guided image-to-image translation, CVPR oral 2021 (https://github.com/cv-rits/CoMoGAN)
[2] F. Pizzati et al., Model-based occlusion disentanglement for image-to-image translation, ECCV 2020
[3] F. Pizzati et al., Physics-informed guided disentanglement in generative networks, under peer review
Bio: Fabio Pizzati is a third-year PhD student at Inria Paris, in the ASTRA team (previously RITS). He is supervised by Raoul de Charette. His research interests lay in the intersections of generative networks, computer graphics, and physics.
Homepage: fabvio.github.io
Sponsored in part by: Meta Reality Labs Pittsburgh