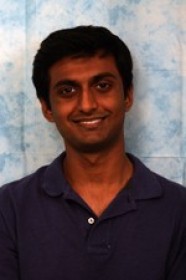
11:00 am to 12:00 am
Event Location: GHC 8102
Abstract: Fast and accurate human pose estimation enables a wide spectrum of applications from interactive control, markerless motion capture and gesture recognition to providing rich semantic information for higher level vision tasks such as scene understanding. The goal of this work is to develop an accurate and real-time human pose estimation system that operates on monocular images from commodity RGB cameras.
Human pose estimation is challenging due to the large number of possible configurations of the underlying articulated skeleton as well as the large variation in the appearance of humans. A core characteristic of the human pose estimation problem is the trade-off between designing an expressive scoring function to evaluate these configurations and the computational complexity of finding the best configuration. In this work, we develop methods for detection, tracking and reconstruction of human pose that incorporate expressive modeling constraints while remaining computationally efficient.
Current systems for human pose estimation from natural images are still far from being deployed in the field. The main impediments to improving pose estimation accuracy and performance are the lack of training data and the need for computationally efficient, yet expressive modeling. In proposed work we intend to tackle these challenges in four ways: (1) by using semi-supervised methods to leverage large amounts of unlabeled video of people (2) collecting data covering large pose variation using a large multiview camera array (3) extending the pose machine architecture to incorporate additional multiscale and temporal cues and (4) exploiting the highly parallelizable nature of the developed pose machine architectures.
Committee:Yaser Sheikh, Co-chair
Takeo Kanade, Co-chair
J. Andrew Bagnell
Andrew W. Fitzgibbon, Microsoft Research, Cambridge
Deva Ramanan, University of California, Irvine