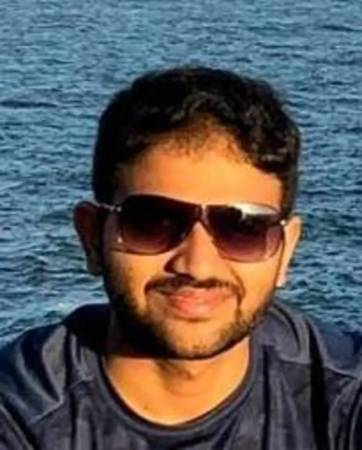
3:00 pm to 4:00 pm
GHC 6501
First, I will talk about my research on visual navigation, which develops predictive representations that enable a robot to anticipate the presence of free space, obstacles, and objects for exploration and object search in novel environments. Predictive representations promote cost-effective learning and result in efficient and performant navigation policies for simulated and real-world environments. Next, I will discuss my latest work on developing efficient and accurate episodic systems for long-form egocentric videos. My research proposes inexpensive predictive representations that capture the coarse context of rooms, objects, and interactions in the video and develops a clip-sampling policy that anticipates the relevant subsets of the video to answer a given human query. The resulting EM system is highly efficient during inference (4-10x lower cost) and performs comparably to the state-of-the-art. Finally, I will discuss the future directions stemming from my research.
Bio:
Santhosh Kumar Ramakrishnan is a Ph.D. candidate in the Department of Computer Science at the University of Texas at Austin, advised by Dr. Kristen Grauman. His research in computer vision and machine learning focuses on egocentric video understanding and visual navigation for robotics. He has authored or coauthored research articles at top computer vision and machine learning conferences such as CVPR, ECCV, NeurIPS, ICLR, and ICML. His research has been selected as oral presentations at CVPR, a spotlight at ECCV, and featured on the magazine cover of Science Robotics. He has also won multiple outstanding reviewer awards at top conferences.
Homepage: Santhosh Kumar Ramakrishnan (srama2512.github.io)
Sponsored in part by: Meta Reality Labs Pittsburgh