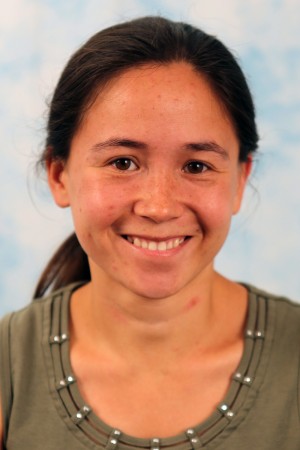
Abstract:
Robotic systems often require that tradeoffs be made–for example, between performance and robustness, power and longevity, or efficiency and safety. While roboticists can design cost functions with hand-picked weights for different metrics, it is not always a straightforward task, particularly when some aspects of performance are not easily quantified. This can occur especially when different combinations of costs result in unexpected emergent behavior, or when performance is based primarily on how humans perceive the robot, e.g. user comfort, social appropriateness, or trust. In line with other recent work on learning from user preferences, we would like to be able to enable users to personalize these performance tradeoffs, particularly when no quantifiable measure exists.
In this proposal, we discuss aspects of safety and comfort in the context of guide robots for indoor navigation, and present both guide robots and assistive robotics as a whole as an exemplar domain where personalization of performance metrics is necessary. We then demonstrate the efficacy of a black-box optimization method for tuning a social navigation algorithm via user preferences. We propose to extend this optimization technique to learn from multiple sources and model multiple objectives; then, using this method, study cost personalization and communication in the context of human-robot teams.
Thesis Committee Members:
Aaron Steinfeld, Chair
Changliu Liu
Andrea Bajcsy
Laurel Riek, UC San Diego