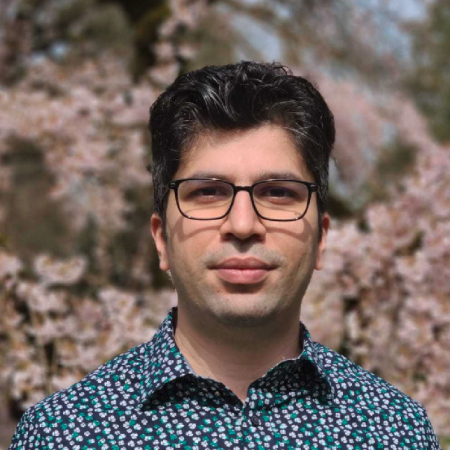
Abstract:
There is a growing interest in using data-driven methods to scale up manipulation capabilities of robots for handling a large variety of objects. Many of these methods are oblivious to the notion of objects and they learn monolithic policies from the whole scene in image space. As a result, they don’t generalize well to different scenes, viewpoints, and lighting changes. In addition, these models cannot be combined with other components and constraints without re-training. In this talk, I will present our approach for learning object centric models trained on 3D depth data. I will show how these approaches are combined with each other to accomplish tasks on unseen objects and environments. In particular, I will cover our works on grasping and segmenting unknown objects, obstacle avoidance, and task planning for unknown object rearrangement task.
BIO:
Arsalan Mousavian is a Senior Robotics Research Scientist at NVIDIA. Prior to NVIDIA, He earned his Ph.D. in computer science from George Mason University in 2018 and Masters degree in Robotics and Artificial Intelligence from University of Tehran in 2013. Arsalan’s research focuses on robotics and 3D computer vision. He is interested in using deep learning, 3D geometry, and classical robotics algorithms to develop robot object manipulation algorithms that can deal with unknown objects in previously unseen environments. Arsalan has published papers in top robotics and computer vision conferences such as RSS, ICRA, CoRL, CVPR, and ICCV including Robot Manipulation Paper Finalist at ICRA 2020.
Sponsored in part by: Facebook Reality Labs Pittsburgh