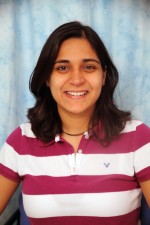
2:00 pm to 3:00 pm
GHC 4405
Abstract:
The goal of this dissertation is to develop computational models for robots to detect and sustain the spatial patterns of behavior that naturally emerge during free-standing group conversations with people. These capabilities have often been overlooked by the Human-Robot Interaction (HRI) community, but they are essential for robots to appropriately interact with and around people in many human environments.
In this work, we first develop a robotic platform for studying human-robot interactions, and contribute new experimental protocols to investigate group conversations with robots. The studies that we conducted with these protocols examine various aspects of these interactions, and experimentally validate the idea that people tend to establish spatial formations typical of human conversations with robots. These formations emerge as the members of the interaction cooperate to sustain a single focus of attention. They maximize their opportunities for monitoring one another’s mutual perceptions during conversations.
Second, we introduce a general framework to track the lower-body orientation of free-standing people in human environments and to detect their conversational groups based on their spatial behavior. This framework takes advantage of the mutual dependency between the two problems. Lower-body orientation is a key descriptor of spatial behavior and, thus, can help detect group conversations. Meanwhile, knowing the location of group conversations can help estimate people’s lower-body orientation, because these interactions often bias human spatial behavior. We evaluate this framework in a public computer vision benchmark for group detection, and show how it can be used to estimate the members of a robot’s group conversation in real-time.
Third, we study how robots should orient with respect to a group conversation to cooperate to sustain the spatial arrangements typical of these interactions. To this end, we conduct an experiment to study the effects of varied orientation and gaze behaviors for robots during social conversations. Our results reinforce the importance of communicative motion behavior for robots, and suggest that their body and gaze behaviors should be designed and controlled jointly, rather than independently of each other. We then show in simulation that it is possible to use reinforcement learning techniques to generate socially appropriate orientation behavior for robots during group conversations. These techniques reduce the amount of engineering required to enable robots to sustain spatial formations typical of conversations while communicating attentiveness to the focus of attention of the interaction.
Overall, our efforts show that reasoning about spatial patterns of behavior is useful for robots. This reasoning can help with perception tasks as well as generating appropriate robot behavior during social group conversations.
Thesis Committee Members:
Aaron Steinfeld, Co-chair
Scott E. Hudson, Co-chair
Kris Kitani
Brian Scassellati, Yale University