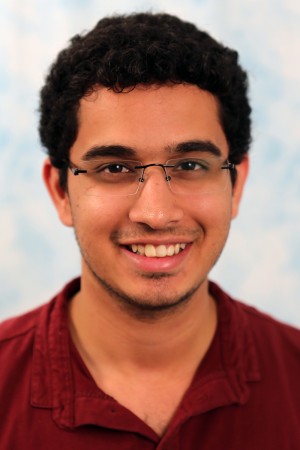
Abstract:
Achieving real-world robot safety requires more than avoiding risk—it demands embracing and managing it effectively. This thesis presents a safety case for risk-aware decision-making and behavior modeling in complex, multi-agent environments such as aviation and autonomous driving. We argue that safety arises from an agent’s ability to anticipate uncertainty, reason about intent, and act within operational boundaries informed by prior knowledge, rules of the road, social context, and historical precedent.
To enable safe and interpretable decision-making, we integrate Monte Carlo Tree Search with formal logic specifications to improve rule adherence in learned policies across both single- and multi-agent settings. We develop symbolic rule-mining techniques using inductive logic programming, distilling interpretable constraints from routine trajectories and real-world crash reports. To handle out-of-distribution risk, we propose a fusion framework that combines neural imitation learning with symbolic rule-based systems. To mitigate modeling risks in complex environments, we explore retrieval-augmented generation (RAG) using crash reports to support grounded action arbitration.
To support learning from real-world behavior in aviation, we introduce three datasets: TrajAir, a social aerial navigation dataset; TartanAviation, a time-synchronized multimodal dataset for intent inference; and Amelia-48, a large-scale airport surface movement dataset spanning 48 U.S. airports, enabling predictive analytics for air traffic management.
Together, these contributions and the tools developed along the way enable autonomous systems to reason under uncertainty, incorporate diverse priors, and operate reliably in complex, real-world settings.
Thesis Committee Members:
Sebastian Scherer (Chair)
Jean Oh
Andrea Bajcsy
Hamsa Balakrishnan (Massachusetts Institute of Technology)