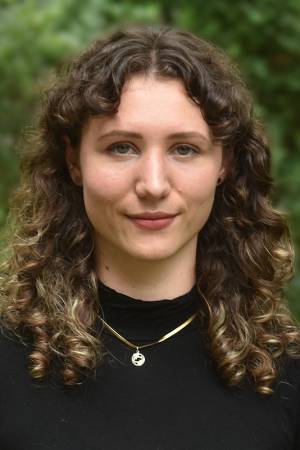
2:30 pm to 3:30 pm
1403 Tepper School Building
Abstract:
Robot safety is a nuanced concept. We commonly equate safety with collision-avoidance, but in complex, real-world environments (i.e., the “open world’’) it can be much more: for example, a mobile manipulator should understand when it is not confident about a requested task, that areas roped off by caution tape should never be breached, and that objects should be gently pulled from clutter to prevent falling. However, designing robots that have such a nuanced safety understanding—and can reliably generate appropriate actions—is an outstanding challenge.In this talk, I will describe my group’s work on systematically uniting modern machine learning models (such as large vision-language models and latent world models) with classical formulations of safety in the control literature to generalize safe robot decision-making to increasingly open world interactions. Throughout the talk, I will present experimental instantiations of these ideas in domains like vision-based navigation and robotic manipulation.
Bio:
Andrea Bajcsy is an Assistant Professor in the Robotics Institute at Carnegie Mellon University where she leads the Interactive and Trustworthy Robotics Lab (Intent Lab). She broadly works at the intersection of robotics, machine learning, control theory, and human-AI interaction. Prior to joining CMU, Andrea received her Ph.D. in Electrical Engineering & Computer Science from University of California, Berkeley in 2022. She is the recipient of the Google Research Scholar Award (2024), Rising Stars in EECS Award (2021), Honorable Mention for the T-RO Best Paper Award (2020), NSF Graduate Research Fellowship (2016), and worked at NVIDIA Research for Autonomous Driving.